Update (21/06/2022): On June 9th, the Bank of Canada released its Financial System Review, which highlighted certain risks of a rapid rise in mortgage costs and contains excellent data. The Bank of Canada policy rate has increased by 50 basis points since this article was written. On June 21, the Globe and Mail published a front-page article titled “Why housing costs are driving up inflation, despite the real-estate downturn” which draws several of the same conclusions as I’ve outlined in this article.
Now that you know what right-half-plane zeros are, in this article I'm going to begin a deep-dive into a control-systems-based analysis of a certain feedback system that is drawing particular public attention today: inflation.
Specifically, shelter inflation. Starting with Canada.
The overarching theme of These Are Systems is that a system is a system no matter what it’s made of, so let’s treat it like one and see what we can learn. The idea of applying systems theory to economics is not the dominant paradigm today, but neither is it new. So let's take a look.
A bit of preamble
I was motivated to write this piece after noticing a deep and startling disconnect between my own systems-based interpretation of the data, and the narratives that I was observing in the public sphere and most macroeconomic forecasts.
As this is a deep-dive article, the level of mathematical detail will be higher than some other articles; however, I aim to keep the logic and conclusions clear and easy to follow even for readers that aren't interested in following along with the math.
This subject bridges from the control systems engineering to a rather more hotly debated topic of macroeconomics, where there are competing schools of thought with widespread disagreement between experts. And any topic that overlaps with public policy inevitably develops a political interpretation as well. I do not intend with this article to support any political party or viewpoint.
I aim to build a picture in concrete steps while citing my assumptions, but for the sake of brevity and maintaining focus, I will be using some foundational concepts that I haven't yet explained in detail. I hope to have the opportunity to explain them in future articles.
I am neither an economist nor a financial analyst. Nor is this an econ blog. I am an engineer, and nothing I write here should be interpreted as financial advice. With this article, I merely aim to help provide you with an effective and predictive mindset and some insight into how concepts from control theory can be applied in practice to real-world systems. It is up to you to choose how you apply this mindset.
The Canada situation
Between August 2020 and August of 2021, the average price of a house in Canada grew by somewhere in the range of 18.4%~21.3%, depending on how you measure. Homebuyers were taking out larger and larger mortgages to afford a home. And yet, over the same timeframe, the cost of paying interest on those mortgages fell by 9.3%.
But Canadian housing still had a long way to grow. By March 2022 the Teranet national home price index once again recorded year-over-year growth of 18.4%.
At the risk of understatement, this isn’t an entirely new trend in Canada.
And yet, consider a related notion: the cost of shelter. This measures not the cost of purchasing a house, but rather the cost of living in a house: paying rent if you rent, paying your mortgage if you have a mortgage, paying for repairs, and so on.
Despite the remarkable increases in the prices of Canadian real estate over the past thirty years, the cost of shelter, has been increasing at a remarkably unremarkable pace:
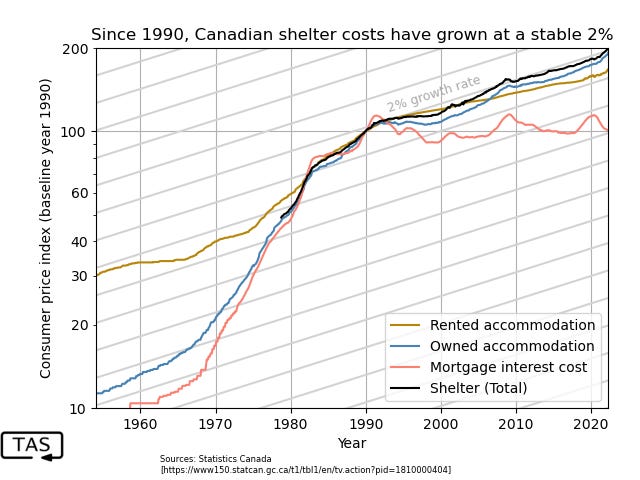
You can see two clear regimes in this graph:
Pre-1990: Rapid (~10%) shelter price growth, pulled upward by mortgage interest costs that were growing at up to 25% per year;
Post-1990: Stable 2% shelter price growth, with mortgage interest costs being absolutely constant outside of some short-term fluctuations.
Now let’s overlay this with house prices, which are excluded from the consumer price index. I’ve drawn from three published housing price sources.
Take a minute to appreciate the sheer magnitude of this uninterrupted growth.1 Even on a log scale, I had to stretch the graph to match the previous chart’s scaling. But the point isn’t just that Canadian housing prices are high, which everyone knows already.
Since 1990, the price of a residence has increased by 490% (nearly all post-2000).
Over that same 32-year period, the cost of shelter merely doubled.
And the cost of a mortgage to pay for that house hasn’t changed at all.
A house is the asset that provides shelter. How is it possible to have such a sustained decoupling between the price of a house and the price of being housed?2
Declining mortgage interest rates is the answer:
But look closely: you’ll notice that mortgage costs kept growing ten years after interest rates peaked in 1981. This is not a coincidence, and is in fact a huge challenge for policymakers attempting to respond to shelter inflation. This post will examine why that is.
And this shapes my central challenges for this deep-dive series:
What are the conditions that allow this divergence to continue?
What will happen if those conditions are not sustained?
Can we model this using control theory, to make predictions?
What consequences for system stability can we learn from that model?
The impact of shelter costs on broader inflation is going to be a big part of that, especially when we close the loop in a future deep-dive and see how inflation impacts interest rates.
How to understand a system
Let me just take a step back and show the big picture, since this is the first time we’re doing this in a deep-dive, and some of the readers of this article might not be control engineers.
Feedback systems can be a real tangled mess, where cause and effect can be hard to distinguish.
The way that we figure out how a system works is by breaking it down into the simplest building blocks that we do understand. Each block, or sub-system, is a little machine that takes in some input signals, and produces some output signals, according to whatever laws govern that block. Like a thermostat, an air conditioner, and an indoor space. We first think about each piece in isolation.
From the point of view of each sub-system, its inputs are just taken as a given, without regard to where they came from.
Then, we’ll connect those blocks together to build a more complete system. As long we just hook up this chain one way, from input to output, it’s called an “open loop" system.
Finally, to figure out how the system really behaves, we close the loop: connecting any outputs back to any matching inputs, so they’re no longer “taken as given”, but generated by the system itself as it runs.
The sub-systems can be linear, nonlinear, random, or anything else. The one requirement is causality: each sub-system needs to be able to generate its output using only observations from the past. For example, whatever determines today’s rent prices has to depend only on the observed data that exists today. That could include predictions about what the data might be tomorrow, but not the actual future values.
By the way, once we’ve pieced this whole thing together, we can treat the whole thing (feedback loop included) as another building block to assemble and more complex systems with it.
This is the strategy that we will use to understand the dynamics of shelter inflation.
Background: The Consumer Price Index in Canada
Some of the readers might not be economists either. If you’re not familiar with how the consumer price index measures inflation, here is a brief explainer.
The term inflation usually refers specifically to consumer price inflation, and is defined as the rate of increase of the average price of consumer goods across the economy.
There is no single consumer good, and no objective "average price", so in practice inflation is approximated using a metric known as the Consumer Price Index (CPI), which aggregates the prices of a "basket" of goods and tracks the change of the price of that basket over time.
The basket weighs the relative influence of various categories of goods, and those weights can change over time.
There are also additional metrics derived from the CPI, known as core inflation, which exclude certain high-volatility components in the hopes of being more predictive.
When it comes to shelter, the consumer price index aims to track how much a house costs as a consumer expense. It deliberately excludes how much the house is worth as an asset.
In Canada, the consumer price indices are tracked by Statistics Canada.
In 2020, shelter made up about 30% of the Canadian CPI basket, making it the largest category by nearly double the runner-up (food, at about 16%, which has volatile prices and is frequently excluded from core CPI). Statistics Canada divides shelter into rented accommodation (for renters), owned accommodation costs (for homeowners), and finally costs of domestic water/fuel/electricity.
Critically, the Canadian category of “owned accommodation” does not use the American method of owner’s equivalent rent, instead preferring to track the cost of already owning a home, e.g. the cost of upkeep, property taxes, insurance, and yes, mortgage interest payments.
In both Canada and the United States, shelter is such a heavily-weighted category that it would dominate inflation metrics if not for the fact that shelter costs tend to change quite gradually.
In fact, even the CPI as a whole tracks shelter costs quite closely, even after subtracting the shelter category itself.
This isn’t likely by chance, which suggests that at least one of the following is probably at play:
Some part of shelter costs are caused by non-shelter inflation factors, eg. the cost of repairing a house scales with the cost of lumber and carpenter-hours
Non-shelter inflation factors are caused by shelter costs, eg. carpenters have to charge more per hour when their rent goes up
Both shelter and non-shelter inflation are influenced by a common root cause, eg. a baby boom that creates a bunch of demand for living space at the same time as it creates a bunch of demand for baby food and diapers.
Finally, I’ll briefly mention that the accuracy of the CPI as an indicator of true cost-of-living has been widely criticized. But agree or disagree with it, the CPI is one of the main feedback signals that steer the ship, and that is what makes it topical to These Are Systems. So I’ll be taking it as it is.
Building a systems model for shelter
We’ve just established that CPI does not measure housing prices, because they’re an asset. But that doesn’t mean that we can just disregard them entirely! They’re still an important input that influences the shelter prices that do show up in the CPI, even though not directly.
Let’s build a simple simulation that lets us better understand precisely how.
Here’s a high-level diagram of our shelter system. Let’s see if we can piece together some equations to turn this into a concrete thing we can poke and play with.
Let’s go through this, item by item.
1. Mortgage interest payments
Given housing prices and interest rates as inputs, we should be able to estimate the resulting mortgage payments.
Mortgage costs are determined in a straightforward way by the house purchase price and the mortgage rates: when you buy a house, you sign a mortgage contract.
But most people who are paying a mortgage this year didn’t buy a house this year. They could have bought it up to 25 years ago.3
As such, we want to average over all outstanding mortgage contracts today. That should include a weighted average over house prices; a house bought 20 years ago might be mostly paid off by now. Mortgage rates should be averaged too, but over a shorter timeframe as Canadian mortgages are essentially 1 to 5 year contracts.4
So this is our mortgage payments subsystem model; pretty simple:
As you saw there’s a lot of different mortgage rates (each dataset over a different span of time), so I’ve just averaged them together to create one effective average mortgage rate.
The results are surprisingly close for such a basic model.
But maybe that shouldn’t be surprising, because it’s basically the method Statistics Canada uses to calculate average mortgage costs.
Let’s put this simple model to work. If Canadian housing prices and mortgage rates were to both freeze exactly where they are, what would mortgage costs do over the next five years?5 It’s a more interesting question than it seems, so think for a second before you scroll down.
Last chance to come up with your own answer!
OK. The answer is that mortgage costs would grow rapidly for five years before gradually levelling off.
I don’t want this to be a subtle point. We’ve hardly even assumed anything in our model yet; it’s just basic accounting so far. The scenario even disregards the policy announcement in June that is widely expected to be another 50 basis point hike. Much of the rest of this post will be exploring the ramifications of this basic idea.
Why does this happen? It’s because each year the older mortgages, which were issued when housing prices and interest rates were lower, drop out of our running average and get replaced by mortgages at the new interest rate.
And by the way, this is already fated — even an immediate housing crash isn’t enough to stop this trend. If housing prices were to suddenly drop by half in 2022 (which would be much more than US prices corrected in 2008), and then permanently ceased growing, mortgage costs would still grow at 8.5% over the next five years. This is a big ship that steers very slowly.
In fact, the scenario that keeps mortgage costs constant at their existing level — the trend of the past 32 years — would require the average available mortgage rates to continue falling below 1% by 2027 if house prices flatline at today’s level.
A key takeaway is that, while housing prices aren’t included in inflation measurements, the accumulated asset price growth will eventually show up in shelter inflation. This happens when interest rates stop decreasing, and even moreso if they rise.
By the way, I’m not claiming that any of these particular scenarios are likely. They’re not necessarily even self-consistent: an aggressive rate cut would almost definitely result in housing prices growing much higher, rather than flat-lining. Nor have we even simulated any rising-rate scenarios. So these are not predictions, just “if-then” scenarios run with the inputs taken as given, to explore the consequences of this system behaving the way we think it does.
To make actual predictions, we’d need a model that also estimates future housing prices and interest rates. We’ll get there.
2. Homeowner upkeep costs
We’ve now modelled mortgage interest costs, but that only represents 10%~20% of the shelter basket, and 18%~25% of the cost of owned accommodation in the CPI.
Having split out the interest payments, what’s left is:
Homeowners’ replacement cost (ie, cost of construction)
Property taxes and other special charges
Home and mortgage insurance
Maintenance and repairs
Other owned accommodation expenses
These basically all scale with the cost of labour and materials. I don’t think there are any specifically interesting system dynamics worth considering here, so we can just consider all of these as trending with broader ex-shelter inflation, without creating a more detailed model.
I’ll spare you the details but the factor of 0.245 scales the home upkeep costs to be the correct ratio with mortgages per the 1992 basket weights (which I chose as the closest basket to my 1990 baseline).6
This model underestimates owned accommodation costs midcentury and trails a little bit post-2000s, but otherwise is pretty close. We’re not aiming for perfection here, and this is plenty accurate for our purposes.
3. Rent
Rent is less straightforward, so we’re going to have to take more of a leap.
Rent is mostly determined by market forces — the balance of supply and demand — although the existence of rent control means that there will always be a population of renters that are paying rents that were locked in at rates no longer available on the market.
There should be something of a link between the cost of renting and the cost of buying a home, because if renting became vastly cheaper than owning a house, fewer people would want to own houses and buying it would get cheaper. Likewise, if renting became vastly more expensive than owning a house, more renters would move to buy houses, driving up the cost of homeownership.
Looking back at our first graphs, we saw that rent grew much slower than inflation before 1980, grew in-line with inflation (and the rest of shelter) from 1980 to 1990, and grew slower than inflation after 1990 (at about 1% per year). I’ll repeat the plot here, adjusting for inflation to make the trend clear. Inflation-adjusted, rents peaked in 1956 and have been declining ever since.
The low rent inflation from 1950 through the 1970s is perplexing, especially since there was never an accelerated period of catch-up to compensate. This means that in relative-to-income terms, either the average rental is extremely cheap today, or else it was extremely expensive to be a renter in the ‘50s. And I don’t think it’s cheap today.
And by the way, the fraction of renters has hardly changed in Canada. (That also makes the math easier, and so I’ll be treating it as a constant.)
So, why?
I wasn’t surprised that rent inflation would be slow post-1990, as falling interest rates and rapid capital gains meant investors would be satisfied to pay ever high prices for an investment property, even if the rent was barely breaking even.
But by the same logic, a period of rising interest rates like the ‘70s should have pushed rents up relative to housing prices, because an investment property would either need to yield higher cash flow to pay the bills, or else nobody would buy it and its price would fall. This view was recently echoed in the Globe and Mail:
As high as Toronto and Vancouver rents may seem to local tenants, landlords are often losing money on them.
In recent years, many mom-and-pop real estate investors in the two cities have been quietly paying more in mortgage and other ownership costs than they receive in rent, trusting they’d eventually sell at a profit thanks to rapidly rising home values, experts say.
But as interest rates shoot up and price growth slows, some highly indebted landlords are beginning to feel the squeeze more acutely. That financial pain could eventually push rents even higher, some experts warn.
After a great deal of research, I’m still not entirely sure why 1970s rental inflation was so low when fundamentals should have been pushed rent up relative to housing prices.
There are good papers by the US Federal Reserve and the Reserve Bank of Australia that look to empirically model these relationships very accurately. These are very good approaches. My approach will be similar in nature but simpler. I am aiming to build a very rough causal model with as few adjustable parameters as I can get away with, because when feedback loops are at play, it can be hard to separate cause from effect.
To estimate rent from inputs like price, let’s first flip this around and calculate: what price should an investor be willing to pay to acquire a rental property? Once we know that, we can work backwards from price (taken as given) to predict the corresponding rent.
In a given year, the income earned by a rental property is:
annual income = rent + capital gain - ownership cost
The amount an investor should be willing to pay up-front to own an income stream is roughly:
price = annual income / discount rate
Seeing as banks are willing to offer loans to buy property at the rate of a mortgage, rental property investors will probably also have a discount rate similar to the mortgage rate. So when we solve for the annual rent an investor should want for a property of a given price:
rent = (price * mortgage rate) + ownership cost - capital gain
Finally we smooth this out, which accounts both for the fact that average rents don’t change on a dime (even when property prices do), and for the fact that things like capital gains are really expectations learned from the average of recent trends. So that gives us our model:
To be clear, I don’t expect this to nearly capture all the intricate complexities of the rental market (nor Statistics Canada’s quality adjustments). This will be a much more approximate fit than our owned accommodation model, but I think it captures the fundamental dynamics.7
… And indeed, this model predicts that rental costs should have increased much more in the 1970s than Statistics Canada says they did. (It mostly suggests that the CPI’s rent in the 1950s was too high for the fundamentals, and property investors should have been bidding up rentals for the cash flow). Was this all due to quality adjustments? I would be curious to hear from those who were in the rental or investment property markets at that time, or to find historical rent-to-income ratio data to see if it’s consistent with what the CPI implies.
Putting it all together: Let’s run some scenarios
Earlier we ran some scenarios looking at just mortgage interest, where we saw that even keeping interest rates at their current level leads to very high growth in mortgage interest costs, because the build-up in house prices — which was excluded from the CPI — is quickly transformed into a consumer expense.
But mortgage interest is currently sitting at just about 3% of the CPI, so even a doubling of mortgage costs doesn’t have a huge impact on its own. Now that we’ve put all the shelter pieces together, let’s use the model we’ve built to see the impact on national inflation.
I couldn’t plot all the scenario inputs without complicating the graph, so I’ll explain here:
(Scenario input) — Housing prices flatline at their April 2022 values;
(Scenario input) — For everything in the CPI except shelter, inflation instantly returns to exactly 2% from April 2022 onward and stays there;
(Scenario input) — The available mortgage rate steps to the indicated value and remains there for the decade.
Given these premises, what would be the resulting inflation?
Let me say a few words to help you understand what this image means. Once again, these are not forecasts. This is us feeding controlled “if-then” experiments to the model just to see how our system reacts. The dotted lines are model output for all-items inflation.
This result should illustrate two main points: First, just how much influence shelter has in the CPI, and second, how rapidly $880,000 home asset prices would be converted into shelter consumer prices by 8% mortgage rates.
For reference Scotiabank expects the policy rate to reach 3% by the end of this year, which would probably mean 5-year fixed mortgage rates being offered at about 5.6%. And again, even if that resulted in an overnight drop in housing prices by half, the inflation is mostly already baked in.
And this brings me to the main thesis. Interest rate hikes are the primary monetary policy tool for reducing inflation. But in our graph above, aggressive interest rate hikes correspond to higher inflation scenarios, especially over the first five years following the rate hike.
This is not a contradiction. It is a right-half-plane zero. This is an ice cream system. When you try to move it down, it swerves upward first.
So if you were the central bank, how would you control a system like this? Stay tuned for the next post in this series.
This post isn’t intended as professional engineering advice. If you are looking for professional engineering advice, please contact me with your requirements.
Interestingly, the 6% annual growth rate of housing prices is a pretty stable trend going back to 1935. However, between about 1975 and 2000, that price growth was only enough to keep up with inflation, whereas since 2000, Canadian housing prices have tripled after adjusting for inflation.
I refer to “a house” loosely but I don’t specifically mean single-family housing; the data I use is for national housing indexes which aggregate all housing types.
25-year-amortization mortgages are the most common in Canada, although American readers will be more familiar with 30-year mortgages.
In fact, Canadians could even have bought their home more than 25 years ago, but I’ll neglect HELOCs and reverse mortgages. I don’t think it significantly affects the model, because when you get a HELOC, you borrow against today’s assessed house price, not 1997’s.
This might be surprising to Americans, but in Canada (and many other countries), essentially every mortgage is adjustable-rate. Mortgage products that offer a fixed rate for the entire amortization period of the mortgage are simply not available from lenders. Most Canadians choose between “5-year fixed-rate mortages”, where the rates renew at the market rate each 5 years, or 5-year variable rate contracts, where the interest rate adjusts each year (often with a fixed monthly payment, where an increasing interest rate results in a slower payoff of the principal).
Canadians, on the other hand, may be equally surprised to find out that full-term fixed-rate mortgages are available in the United States, and that what Canadians call a “fixed-rate mortgage” wasn’t the same thing that Americans call a fixed-rate mortgage.
That is, with mortgages frozen at 2.74%, which is the geometric mean of today’s best variable rate (1.9%) and 5-year fixed rate (3.94%) (per ratehub.ca).
A constant scaling factor should actually be a pretty good approximation, even though the basket weights change over time. This is because a constant scaling factor is equivalent to floating basket weights that update as the input magnitudes change, which is what the CPI aims for anyway.
For example, if in 1992 mortgage interest was weighted as 29% of owned accommodation, that should merely reflect a situation where of every dollar a typical homeowner spends on maintaining their house, 29 cents are paid to the bank. If, in the following year, mortgage costs suddenly tripled, the basket weight should update accordingly to reflect its increased importance. And that’s what a constant scaling factor does as well. So it should roughly approximate subsequent changes in the basket weights.
I’ll mention that the CPI weight should also capture changes in purchasing volume, not only price. For example, if the price of coffee doubles in 2025 but consumers entirely switch over to drinking tea, the overall spending on coffee will decrease even though its price has increased, and the CPI basket weights will eventually update to reflect that change. I am making a large, but (I think) well-grounded assumption that this is not very important in the context of shelter:
Almost everyone purchases shelter in one form or another, as it is very hard to live without it;
There aren’t really any substitute goods for shelter like there are for tea and coffee. You can switch between renting or owning, but that’s about it;
The nature of Canadian mortgages should cause volume to change slowly and smoothly. Even if all homebuying and new mortage contract activity immediately halted today, there would be about 20 years’ worth of mortgage contracts already in the system coming up for renewal and sustaining a good fraction of the previous volume. At least for the purpose of predicting the next 5 years, the CPI mortgage interest basket weight should not change in a sudden and drastic way in response to a change in mortgage volumes.
We can test this by comparing the published basket weights that go back to 1986 against the relative growth of each CPI subcategory, under a constant-relative-volume assumption:
And the result of this is… well, I think it’s quite close. There are some discrepancies, but for our purposes, this should be good enough.
Again the rent model requires actual housing price and rent dollar amounts, whereas the CPI data are normalized price indices, so the input magnitudes must be scaled accordingly. I’ve done my best to match these up, but expect qualitative, not quantitative predictions.
How do wages (and thus shelter affordability) figure into these models? Is this simply captured in the inflation figure?
What about I recall hearing stories of the 1970s and earlier that it was harder to get a mortgage than today as banks were more risk-averse in lending to individuals.
I think your missing variable is population growth. The 1950 through 1970 are the peak years of a) the baby boom, and b) post-war immigration. Rental pricing is decided at the margin, and people needed ever-more space through those decades. And the stock of rental changes slowly, with a long delay. https://en.wikipedia.org/wiki/Population_of_Canada#/media/File:Canada_immigration_graph.png